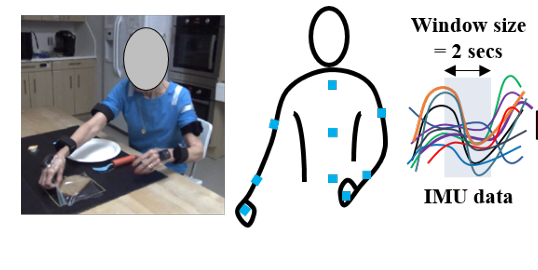
Impairment quantification in stroke patients
The wearable-sensor data and corresponding video features are available here.
This website provides materials relevant to the paper:
Yu, B., Kaku, A., Liu, K., Parnandi, A., Fokas, E., Venkatesan, A., Pandit, N., Ranganath, R., Schambra, H. and Fernandez-Granda, C., Quantifying impairment and disease severity using AI models trained on healthy subjects. npj Digit. Med. 7, 180 (2024).
DOI: https://doi.org/10.1038/s41746-024-01173-x
The paper presents a method to perform automatic assessment of impairment and disease severity using AI models trained only on healthy individuals. The COnfidence-Based chaRacterization of Anomalies (COBRA) score exploits the decrease in confidence of these models when processing data from impaired or diseased patients to quantify their deviation from the healthy population.
This diagram explains how the method quantifies impairment in stroke patients (top) and disease severity of knee osteoarthritis (bottom) :
The plots below compare the COBRA score against expert-based metrics: (a) Fugl-Meyer assessment (FMA) score for impairment quantification in stroke patients, (b) Kellgren-Lawrence (KL) grade for knee impairment. The COBRA score computed automatically in under a minute is strongly correlated with the expert-based metrics.
Yu, B., Kaku, A., Liu, K., Parnandi, A., Fokas, E., Venkatesan, A., Pandit, N., Ranganath, R., Schambra, H. and Fernandez-Granda, C., Quantifying impairment and disease severity using AI models trained on healthy subjects. npj Digit. Med. 7, 180 (2024).
Parnandi, Avinash, et al. "Data-Driven Quantitation of Movement Abnormality after Stroke.” Bioengineering (2023)
Kaku, Aakash, et al. "StrokeRehab: A Benchmark Dataset for Sub-second Action Identification." Advances in Neural Information Processing Systems(NeurIPS 2022)
Parnandi, Avinash, et al. "PrimSeq: A deep learning-based pipeline to quantitate rehabilitation training.” PLOS Digital Health (2022)
Kaku, Aakash, et al. "Towards data-driven stroke rehabilitation via wearable sensors and deep learning." Machine Learning for Healthcare Conference. PMLR, 2020
This work was supported by NIH grant R01 LM013316, Alzheimer’s Association grant AARG-NTF-21-848627, NSF grant NRT-1922658, and NSF CAREER Award 2145542.